Key Insights
NexaStack offers a developer-friendly, enterprise-grade AI deployment environment focused on agentic AI, automation, and cloud-native integration. It emphasises rapid experimentation, edge deployment, and seamless CI/CD for AI workflows. On the other hand, Google’s Vertex AI provides a fully managed, scalable platform with strong MLOps tools and integration within the Google Cloud ecosystem. While Vertex AI excels in model training and deployment at scale, NexaStack stands out for organizations seeking custom agent-based architectures and faster iteration cycles.
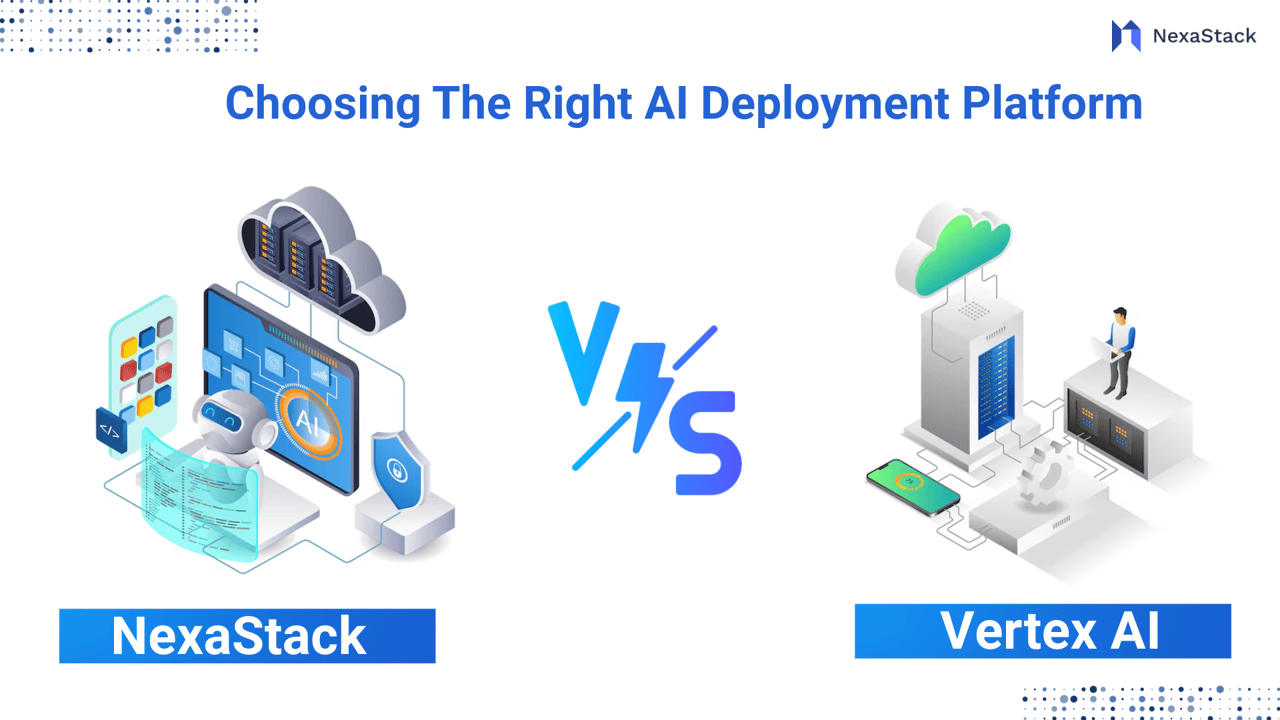
Development Experience and MLOps Integration
A seamless development experience and robust MLOps capabilities are essential for accelerating AI model deployment and ensuring operational efficiency.
Model Training, Experimentation, and Deployment Workflows
Efficient model training, experimentation, and deployment processes help streamline AI lifecycle management.
-
NexaStack offers a containerised AI development environment with Jupyter Notebooks and supports custom ML pipelines for flexible experimentation.
-
Vertex AI integrates seamlessly with Google AI Platform Notebooks and Kubeflow Pipelines, enabling a cloud-native approach to AI development.
CI/CD Pipeline Integration and Version Control
Continuous integration and deployment (CI/CD) enhance AI model reliability by automating testing and versioning.
Both platforms provide:
-
Model versioning and rollback mechanisms for safe experimentation.
-
CI/CD pipelines to automate AI model deployment and updates.
-
Automated model drift detection to ensure continuous model accuracy.
Monitoring, Observability, and Model Governance Features
Real-time monitoring and governance help maintain AI model performance and compliance.
-
NexaStack provides customizable monitoring dashboards with built-in anomaly detection for proactive issue resolution.
-
Vertex AI leverages Google Cloud Logging and AI Explainability tools to enhance model observability and transparency.
Strategic Decision Framework and Implementation Roadmap
Selecting the right AI platform is a structured process involving business goals, infrastructure, and regulatory requirements.
Key Evaluation Criteria for Platform Selection
Companies need to consider various factors before deciding between NexaStack and Vertex AI:
-
Scalability & Flexibility: Will the platform scale to support future AI workloads and hybrid/cloud deployments?
-
Security & Compliance: Does it cover industry-specific needs like GDPR, HIPAA, or SOC 2?
-
Cost & Resource Optimisation: How does the pricing model impact long-term ROI and operating expenses?
Implementation Timeline and Resource Planning Guide
Deployment schedules vary with migration complexity and infrastructure
-
NexaStack: Ideal for hybrid/on-prem, deployable in weeks with existing IT infrastructure integrations.
-
Vertex AI: Cloud-first deployment is possible in days, but entails Google Cloud transformation and migration.
Future-Proofing Your AI Infrastructure Investment
To become long-term sustainable, businesses must select platforms that adapt to AI innovation:
-
LLMS, Generative AI, and MLOps automation enhance model lifecycle efficiency.
-
AI model explainability and responsible AI frameworks ensure responsible AI adoption and regulation compliance.