Key Insights
Compound AI Systems combine multiple specialised AI models or agents to solve complex tasks collaboratively. By coordinating diverse capabilities like reasoning and perception, they offer greater adaptability, scalability, and resilience, shaping the future of more intelligent and dynamic AI ecosystems.
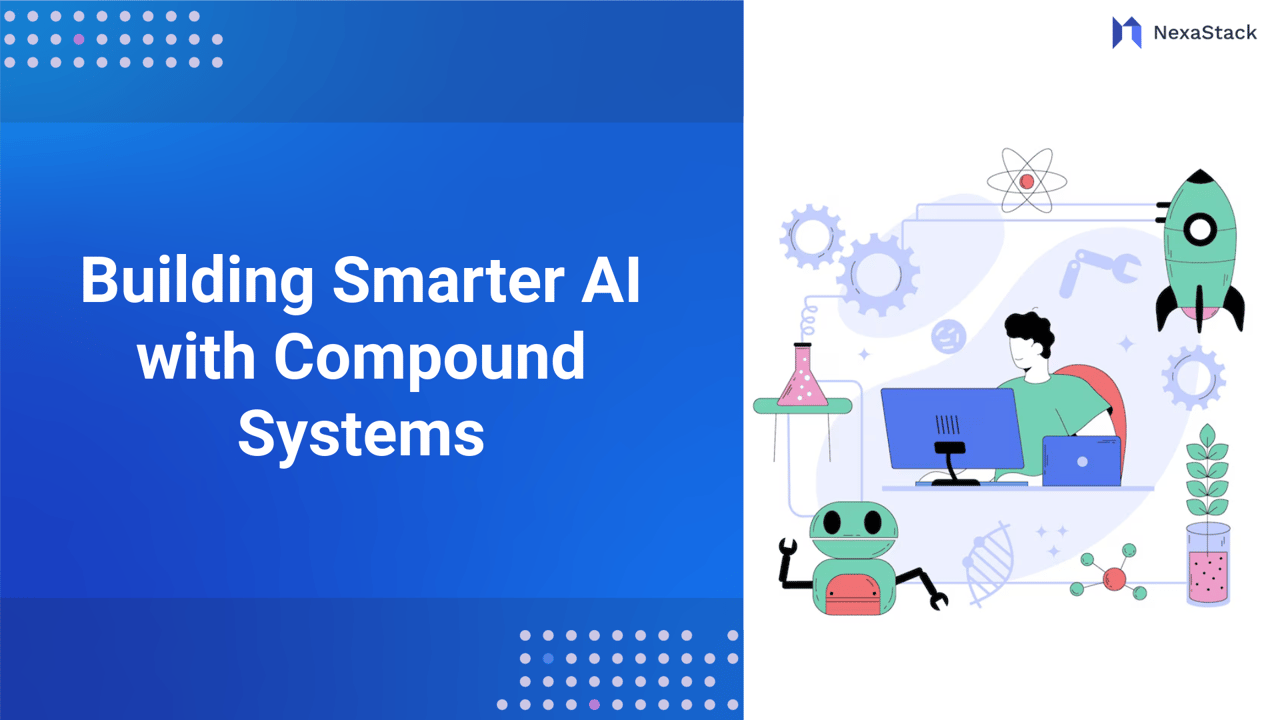
Businesses operating in the current period of fast-paced digital change and expanding AI technology adoption search for more efficient ways to unite diverse AI approaches into effective, high-performing systems. Compound AI systems use multiple models to execute business transformation and sustain competitive advantages in the market. The following blog investigates a strategic framework that shows organisations how to develop and implement compound AI systems for large-scale value delivery.
Framework for Designing Multi-Model AI Systems
The design of compound AI systems demands more than combining multiple AI models alone. The process builds synergistic architectural elements to make different system components work together without interruptions. The main goal is optimising system capabilities, which produces superior performance that the models would achieve separately. The structured approach contains multiple essential components to achieve this goal.
Strategic Value Assessment
This initial phase starts the strategic value assessment of compound AI systems. Organisations need to evaluate their existing performance compared to their business problems while assessing multi-model AI solutions for potential solutions. Multiple stakeholders representing various departments must be part of this assessment to obtain a whole business perspective.
The strategic value assessment requires specific essential elements to be considered.
-
The strategic value assessment requires specific essential elements to be considered.
-
The assessment should focus on discovering optimisation chances combined with automation to build operational efficiency and minimise costs.
-
Organisations should assess the present data and infrastructure systems to evaluate their ability to integrate compound AI solutions.
-
Analysts generate quantitative and qualitative findings that predict revenue effects, cost savings, and improved customer experiences as part of the assessment process.
-
Business goals receive support from AI initiatives through vision statement development and strategic roadmap creation.
-
Comparative studies must be done to understand how competitors use their equivalent systems.
-
Defining success criteria and performance metrics to gauge the effectiveness of compound AI systems.
Strategic value assessments that cover all aspects help organisations validate their multi-model AI investments through stakeholder-aligned decision-making.
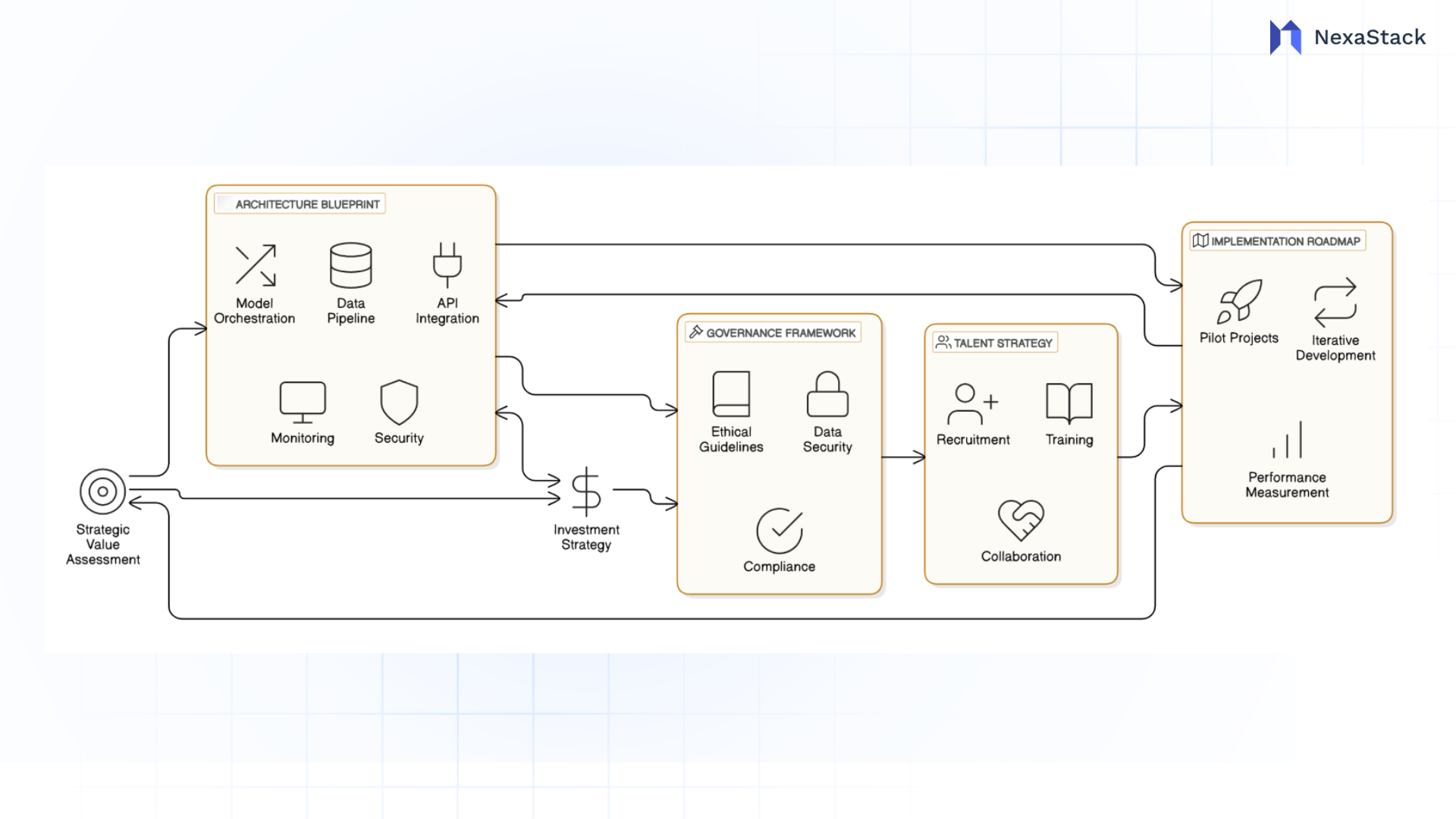
Architecture Blueprint
The following step involves designing an architecture blueprint that should fulfil organisational goals. The developed framework needs to provide smooth connectivity between AI systems and essential business application databases. Improvements should focus on maintaining scalability, flexibility, and robustness.
An architecture blueprint contains essential components, which include:
-
Model Orchestration: The system aligns different models through orchestration to exchange data and process tasks, which delivers performance excellence while reducing response time.
-
Data Pipeline Management: A framework for controlling data processing from start to finish enables guaranteed data quality and consistency with system availability.
-
API Integration: Deploying documented APIS allows business applications to connect with AI models through an interface.
-
Monitoring and Maintenance: A system with alert capabilities should be established to react immediately to performance declines and system failures.
-
Model Versioning and A/B Testing: Through A/B testing and model versioning, organisations can test new algorithm versions alongside running operations for a smooth software update process.
-
Security and Compliance Layer: Ensuring data privacy, protection, and compliance with relevant regulations (e.g., GDPR).
Organisations can achieve business adaptation and new model integration efficiency through modular and scalable system design without requiring full-scale system rebuilds.
Investment Strategy
Financial institutions must develop a detailed plan to spend money on compound AI systems. Organisations must assess system development, deployment, and upkeep expenses relative to estimated profit. A strategy defining investments helps organisations properly distribute their funds and minimise financial risks.
Investment strategy considerations include:
-
Cost-Benefit Analysis: Financial experts use cost-benefit analysis to conduct complete economic assessments of future benefits and present and sustained expenses.
-
Budget Allocation: The organisation should allocate its funds to various projects based on their potential for creating impact, feasibility, and level of organisational readiness.
-
Partnership Models: Building alliances with technology vendors, academic institutions, and AI research organisations to leverage expertise and resources.
-
Risk Mitigation: To mitigate risks, organisations should identify technical, financial, and operational risks and establish contingency measures to protect themselves.
-
Scalability Planning: Business growth predictions will guide the creation of systems that support extended operational capacities.
When executive teams support an investment plan demonstrating responsible financial stewardship, they become more likely to endorse the initiative.
Governance Framework
The governance concept plays an essential role in monitoring compound AI systems. Organisations must establish rules and guidelines to protect responsible use, data privacy, and regulatory standards. Governance guidelines must remain dynamic since they should adjust as technology and laws evolve.
Organisations need to implement three fundamental elements that form a governance framework:
-
Ethical AI Guidelines: Organisations should establish moral guidelines for ensuring responsible AI processes, which maintain fairness, accountability, and transparency.
-
Data Security Protocols: Organisations should implement strong data encryption solutions and access restriction methods to protect sensitive information.
-
Compliance Monitoring: The organisation conducts system audits often to verify that normal data security law requirements and industry standards are followed.
-
Model Auditing and Validation: The continuous procedure of model performance evaluation includes fairness checks to detect biases and model inaccuracies in model auditing and validation operations.
-
Stakeholder Engagement: Active commitment to key stakeholders is part of the framework's decision-making process and policy update governance format.
Installing solid governance standards leads organisations to minimise risks while developing trust in their compound AI solutions.
Talent Strategy
Exceptional capabilities are necessary for constructing and sustaining compound AI systems. Organizations must focus on recruiting, training, and retaining AI experts and data scientists to build their talent strategy. The successful creation of practical solutions depends on active cooperation between domain experts and data scientists.
Talent strategy elements include:
-
Skill Assessment: A skill assessment helps organisations discover skill gaps and build specialised training initiatives to close them.
-
Recruitment Policies: Attracting top talent through competitive compensation, career development opportunities, and a culture of innovation.
-
Collaboration Models: The organisation implements collaboration models that bring together experts, such as data scientists, software engineers, and business analysts, to achieve synergy.
-
Continuous Learning: The team should receive professional development funding to stay informed about recent technologies and methods.
-
Knowledge Sharing: The organisation needs to create internal knowledge repositories and best practice documentation so team members can collaborate effectively.
Organisations that develop diverse talent through skilful staffing can create robust, innovative AI systems that lead to transformative results.
Implementation Roadmap
Successful execution of the strategic vision depends heavily on having specific implementation guidelines that transform the vision into meaningful results. The implementation roadmap for each project section should feature elementary milestones, resource requirements, and defined timeframes. The plan must also contain backup strategies to handle possible barriers during execution.
Roadmap components include:
-
Iterative Development: The development process involves ongoing enhancement of integrated models through repeated model refinement and performance-based feedback evaluation.
-
Performance Metrics: The organization establishes defined KPIs and measurement criteria to assess effectiveness and impact in AI-based operations.
-
Stakeholder Engagement: The organization will involve stakeholders through open dialogue to acquire feedback and maintain their enduring backing.
-
Scalability and Maintenance Plans: As technologies evolve, it is essential to establish maintenance and system update strategies for long-term scalability of systems.
Organizations now use Compound AI systems to lead a significant change in their AI-based innovation approaches. Companies achieve sustainable business transformations through thoughtful multi-model system design, allowing them to discover new growth opportunities. This blog contains a practical guide for building deployable compound AI systems that bring quantifiable benefits to organisations.
Next Steps with Compound AI Systems
Talk to our experts about implementing compound AI system, How Industries and different departments use Agentic Workflows and Decision Intelligence to Become Decision Centric. Utilizes AI to automate and optimize IT support and operations, improving efficiency and responsiveness.